Poro 34B: A 34B Parameter AI Model Trained for 1T Tokens of Finnish, English, and Programming languages, Including 8B Tokens of Finnish-English Translation Pairs
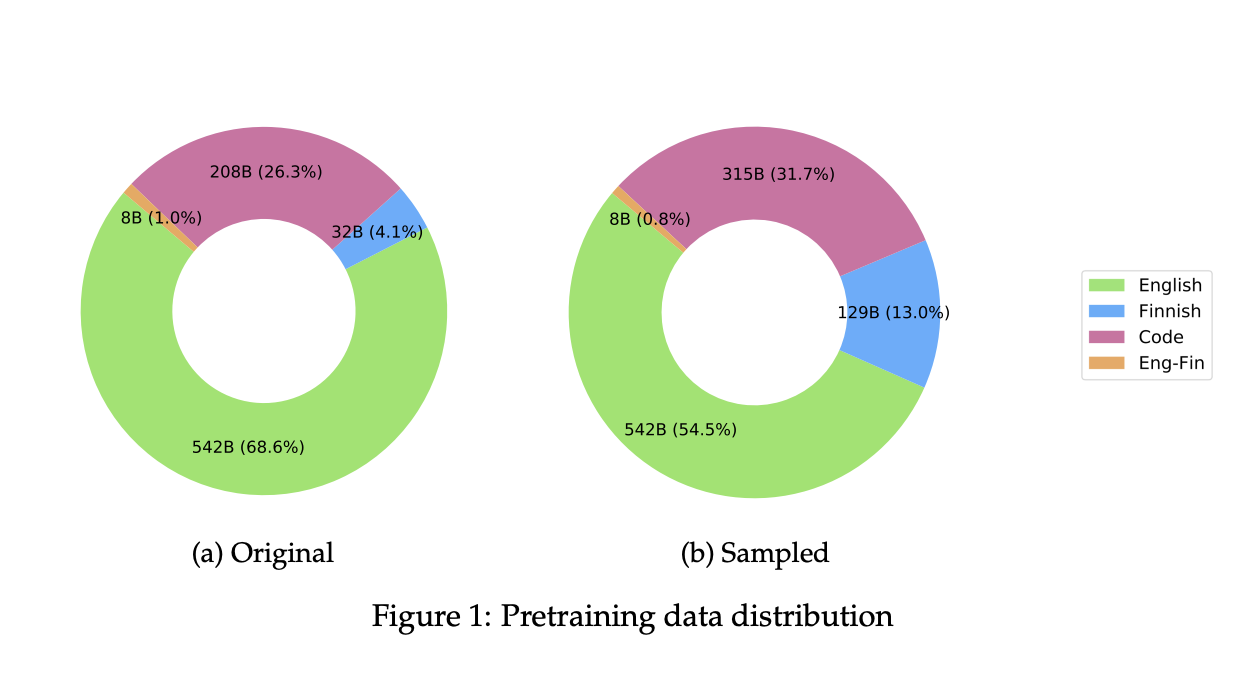
State-of-the-art language models require vast amounts of text data for pretraining, often in the order of trillions of words, which poses a challenge for smaller languages needing more extensive resources. While leveraging multilingual data is a logical solution, it’s commonly viewed as problematic due to the “curse of multilingualism.” Despite some research exploring the benefits and drawbacks of multilingual training and efforts to enhance models for smaller languages, most cutting-edge models still need to be primarily trained in large languages like English. However, there’s potential to significantly improve models for smaller languages through multilingual training, which could mitigate the data scarcity issue.
Researchers from TurkuNLP Group, University of Turku, Silo AI, University of Helsinki, and CSC – IT Center for Science have developed Poro 34B, a 34-billion-parameter model trained on 1 trillion tokens of Finnish, English, and programming languages. They demonstrate that a multilingual training approach significantly enhances the capabilities of existing Finnish models while excelling in translation and remaining competitive in English and programming tasks. By leveraging insights such as limited multilingualism, matching scripts, language families, oversampling, and augmenting with programming language data, they mitigate data limitations and produce state-of-the-art generative models, notably Poro 34B.
For pretraining Poro 34B, the dataset underwent preprocessing to eliminate low-quality and duplicate texts and filter out toxic contexts. Finnish data, sourced from web crawls, news, and Finnish literature, comprises a 32-billion-token corpus, upsampled for four epochs. English data, derived from SlimPajama and Project Gutenberg, amounts to 542 billion tokens, representing over half of the total training tokens. Programming language data, sourced from the Starcoder corpus, is oversampled to represent approximately one-third of the pretraining tokens. A cross-lingual signal is introduced using English-Finnish translation pairs from the Tatoeba challenge dataset, constituting under 1% of the pretraining tokens.
In tokenization, a custom byte-level BPE tokenizer was developed for Poro 34B, with a 128K token vocabulary, aiming for low fertility across Finnish, English, and code. Pretraining involved training the decoder-only model to 1 trillion tokens, surpassing the estimated optimal compute for efficiency. The training utilized a sequence length of 2048 tokens, a cosine learning rate scheduler, and a Megatron-DeepSpeed fork for AMD GPU compatibility. The configuration included 128 nodes, activation checkpointing, and parallelism strategies. Compute cost, estimated at 448MWh, was assessed for environmental impact, considering LUMI’s renewable energy source. Only GPU power consumption was factored into emissions calculation.
The evaluation of the Poro 34B model across multiple dimensions showcases its strong performance. Poro 34B demonstrates low character-level perplexity across English, Finnish, and code, indicating effective learning across these languages. Across various benchmarks, Poro 34B excels, particularly in Finnish tasks, surpassing previous monolingual models. Its English proficiency remains competitive, comparable to models trained predominantly in English. Notably, Poro 34B exhibits commendable coherence and grammatical correctness in open-ended generation tasks in Finnish text generation. Furthermore, its impressive capabilities outperform dedicated translation models and even Google Translate. These results underscore Poro 34B’s versatility and effectiveness across diverse language tasks.
In the study, researchers addressed challenges in training large generative models for smaller languages by developing Poro 34B, a 34B-parameter model trained on 1T tokens of Finnish, English, and code, including 8B tokens of Finnish-English translation pairs. The thorough evaluation revealed significant advancements over existing models for Finnish, competitive performance in English and code tasks, and remarkable translation results. The study highlights the limitations of benchmarks derived from translated tasks. Future research aims to explore the effects of multilingual training systematically. Poro 34B’s release seeks to serve as a template for creating larger models for other smaller languages, facilitating further research and development.
Check out the Paper and HF Page. All credit for this research goes to the researchers of this project. Also, don’t forget to follow us on Twitter. Join our Telegram Channel, Discord Channel, and LinkedIn Group.
If you like our work, you will love our newsletter..
Don’t Forget to join our 39k+ ML SubReddit
Sana Hassan, a consulting intern at Marktechpost and dual-degree student at IIT Madras, is passionate about applying technology and AI to address real-world challenges. With a keen interest in solving practical problems, he brings a fresh perspective to the intersection of AI and real-life solutions.